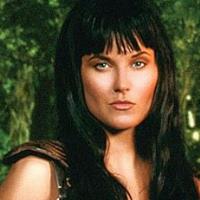
Monday, June 24, 2019 5:57:50 PM
Poster from CTAD 2018:
P146: COMPARISON OF SLEEP MEASUREMENTS FROM
ACTIGRAPHY TO SELF-REPORTED SLEEP DIARIES. Kirsi
Kinnunen1
, Richard Joules1
, Janet Munro1
, Iain Simpson1
, Robin
Wolz1,2, Yves Dauvilliers3 ((1) IXICO Plc, London, UK; (2) Imperial
College London, London - UK; (3) Sleep Unit, Department Neurology,
Centre Hospitalier Universitaire, Montpellier, INSERM 1061 -
France)
Background: Sleep disturbances are common in people living
with dementia. Reduced and fragmented sleep can impact
pathology, worsen other symptoms, and significantly affect
daytime functioning. Additionally, poor sleep quality may
be a risk factor of many neurological conditions, including
Alzheimer’s disease (Ju et al. 2014). While polysomnography
(PSG) remains the gold standard for sleep assessment, it is
costly, often recorded on an isolated night with no daytime
assessment, and may not be feasible in many clinical trials.
The increasing availability of wearable sensor technology
offers a practical, non-intrusive means of collecting continuous
sleep-wake data, over several days. This provides “digital
biomarkers” from real world settings that can be used to
predict risk, detect symptoms and monitor changes in sleep
and activity. Objectives: The aim of this study was to examine
relationships between actigraphy-derived and self-reported
sleep diary measurements within an epidemiology study cohort
of elderly subjects. Methods: We analyzed actigraphy and sleep
diary data, available for 22 subjects (Age: Mean=80.6, SD=9.9;
68% female) from a study of sleep difficulties, lifestyle factors
and general health in the Centre Hospitalier Universitaire,
Montpellier - France. All subjects were asked to wear an Axivity
3-axis accelerometry biosensor device (http://axivity.com/) on
their non-dominant wrist for 14 nights and to complete sleep
diaries for the same 2-week period. IXICO’s fully integrated
wearables work-flow (Figure 1) was employed for data analysis.
This incorporated quality control (QC) for periods of perceived
device “non-wear”. In-bed periods were estimated with the
McVeigh algorithm (McVeigh et al. 2016) and sleep and wake
periods with the Cole-Kripke (CK) algorithm (Cole et al. 1992).
Correlations between the actigraphy- and self-reported sleep
measurements were assessed sample-wise, for all nights with
both types of data available. As part of a quantitative QC,
unrealistic night-time in-bed periods of <2.5 and >13 hours
(N=17) were excluded from subsequent analysis, as well as
self-reported nights with <1 hour of sleep (N=2). This resulted
in 141 nights’ data for the statistical analysis. Correlations
(Pearson’s R/Spearman’s Rho) were calculated between four
variables available from both actigraphy and the diaries: sleep
efficiency/quality, total time in bed, night-time in-bed sleep
and number of awakenings. Results: Actigraphy-derived sleep
efficiency correlated with self-reported sleep quality at r = .16
(p = .06; Figure 2). McVeigh-estimated times in bed at night
and self-reported times from into-bed to out-of-bed correlated
at r = .32 (p = .0001). CK-estimated in-bed sleep times and selfreported times from lights-out to awakening (minus sleep onset
latency) correlated at r = .15 (p = .07). As expected, the number
of estimated night-time awakenings was considerably higher
from actigraphy (median=13, IQR=11) than from the diaries
(median=2, IQR=2), with these measurements correlated at r
= .06 (p = .51). Conclusions: The relatively low correlations
between sleep diaries and actigraphy measures reported here
may implicate poor reliability and inaccuracy of self-reported
sleep measures (see e.g. Goelema et al. 2017). However, as selfreported awakenings may include lying still in bed, the higher
number of actigraphy-derived awakenings may be partially
explained by interpretation of the minute-wise sleep/no-sleep
labeling from the algorithm: a period of wakefulness being
split into several if quiet wakefulness is labelled as asleep. With
sleep efficiency, we have previously shown in the same cohort
that measures derived from 1-night accelerometry recordings
using the CK algorithm (as above) correlated at r = .61 (p =
.003) with simultaneous PSG (Wolz et al. 2017). Using a welldesigned work-flow, actigraphy can provide objective realworld measures of sleep and activity from data collected over 14
days or longer, with minimal patient discomfort and acceptable
QC failure rates. Although PSG can be used to assess sleep
stages, apnea/hypopnea indexes and periodic leg movements,
the advantages of actigraphy over traditional PSG include the
well-tolerated continuous recording in real life settings and
relative cost-effectiveness. The current results suggest that
compared to sleep diaries, actigraphy can offer an attractive
and more reliable alternative for the measurement of signs
and symptoms of disease, or the evaluation of therapeutic
effects. Customized data analytics, including disease-specific
models, have the potential to detect sleep disturbance more
accurately than widely used algorithms such as the CK, which
may incorrectly interpret periods of quiet wakefulness as sleep.
In the Wolz et al. (2017) 1-night study, we found the IXICO
Deep Learning Sleep (DLS) algorithm to outperform the CK
in estimating PSG-derived sleep efficiency (DLS: r = .84, p <
.0001 vs. CK: r = .61, p = .003). Our approach of combining
actigraphy with advanced data analytics shows promise for
providing improved biomarkers of sleep, circadian rhythm
and activity outcomes in clinical trials. In future work, we
will employ the DLS algorithm to estimate sleep efficiency in
elderly populations and neurodegenerative disease cohorts
and will extend the presented statistical analysis to include
daytime naps and an assessment of variability between night
and day activity/sleep over the 14 day period. References:Cole
et al. (1992). Automatic sleep/wake identification from wrist
activity. Sleep, 12(5), 461–9. Goelema et al. (2017). Determinants
of perceived sleep quality in normal sleepers. Behavioural
Sleep Medicine, (20):1-10. Ju, et al. (2014). Sleep and Alzheimer
disease pathology--a bidirectional relationship. Nature Reviews
Neurology, 10(2), 115–9. McVeigh et al. (2016). Validity of an
automated algorithm to identify waking and in-bed wear time
in hip-worn accelerometer data collected with a 24 h wear
protocol in young adults. Physiological Measurement, 37(10),
1636–52. Wolz et al. (2017). Extracting digital biomarkers of
sleep from 3-axis accelerometry using deep learning. The
Journal of Prevention of Alzheimer’s Disease, 4(4), P81.
https://www.ctad-alzheimer.com/files/files/CTAD%20ABSTRACT.pdf
Recent AVXL News
- Anavex Life Sciences to Present at the H.C. Wainwright 26th Annual Global Investment Conference 2024 • GlobeNewswire Inc. • 09/03/2024 11:30:00 AM
- Form 10-Q - Quarterly report [Sections 13 or 15(d)] • Edgar (US Regulatory) • 08/06/2024 09:21:05 PM
- Anavex Life Sciences Reports Fiscal 2024 Third Quarter Financial Results and Provides Business Update • GlobeNewswire Inc. • 08/06/2024 11:30:00 AM
- Anavex Life Sciences to Announce Fiscal 2024 Third Quarter Financial Results on Tuesday, August 6, 2024 • GlobeNewswire Inc. • 08/01/2024 11:30:00 AM
- Form 8-K - Current report • Edgar (US Regulatory) • 07/30/2024 09:20:53 PM
- Anavex Life Sciences Announces Translational Biomarker Data for ANAVEX®2-73 (blarcamesine) in Fragile X Syndrome (Major Cause of Autism) at the 19th NFXF International Fragile X Conference • GlobeNewswire Inc. • 07/30/2024 11:30:00 AM
- Form S-3 - Registration statement under Securities Act of 1933 • Edgar (US Regulatory) • 07/29/2024 09:21:49 PM
- Results from Anavex Life Sciences Landmark Phase IIb/III Trial of Blarcamesine Presented at Alzheimer's Association Conference • GlobeNewswire Inc. • 07/28/2024 09:00:00 PM
- Anavex Life Sciences to Present at the H.C. Wainwright 5th Annual Neuro Perspectives Virtual Conference • GlobeNewswire Inc. • 06/20/2024 11:30:00 AM
- Form 4 - Statement of changes in beneficial ownership of securities • Edgar (US Regulatory) • 06/17/2024 11:30:10 AM
- Anavex Life Sciences Announces Expansion of Leadership Team • GlobeNewswire Inc. • 05/22/2024 11:30:00 AM
- Form 4 - Statement of changes in beneficial ownership of securities • Edgar (US Regulatory) • 05/17/2024 10:01:00 AM
- Anavex Life Sciences to Present at the H.C. Wainwright 2nd BioConnect Investor Conference at NASDAQ • GlobeNewswire Inc. • 05/14/2024 11:30:00 AM
- Shareholders that lost money on Anavex Life Sciences Corporation(AVXL) Urged to Join Class Action - Contact The Gross Law Firm to Learn More • PR Newswire (US) • 05/10/2024 09:45:00 AM
- Form 10-Q - Quarterly report [Sections 13 or 15(d)] • Edgar (US Regulatory) • 05/09/2024 08:35:55 PM
- Form 8-K - Current report • Edgar (US Regulatory) • 05/09/2024 12:00:30 PM
- Anavex Life Sciences Reports Fiscal 2024 Second Quarter Financial Results and Provides Business Update • GlobeNewswire Inc. • 05/09/2024 11:30:00 AM
- The Gross Law Firm Announces the Filing of a Securities Class Action on Behalf of Anavex Life Sciences Corporation(AVXL) Shareholders • PR Newswire (US) • 05/07/2024 09:45:00 AM
- Form DEFA14A - Additional definitive proxy soliciting materials and Rule 14(a)(12) material • Edgar (US Regulatory) • 05/06/2024 10:03:33 AM
- Anavex Life Sciences Corporation Sued for Securities Law Violations - Contact The Gross Law Firm Before May 13, 2024 to Discuss Your Rights - AVXL • PR Newswire (US) • 05/03/2024 09:45:00 AM
- Anavex Life Sciences to Announce Fiscal 2024 Second Quarter Financial Results on Thursday, May 9th, 2024 • GlobeNewswire Inc. • 05/02/2024 11:30:00 AM
- May 13, 2024 Deadline: Contact The Gross Law Firm to Join Class Action Suit Against AVXL • PR Newswire (US) • 04/26/2024 09:45:00 AM
- Contact The Gross Law Firm by May 13, 2024 Deadline to Join Class Action Against Anavex Life Sciences Corporation(AVXL) • PR Newswire (US) • 04/19/2024 09:45:00 AM
- The Gross Law Firm Reminds Shareholders of a Lead Plaintiff Deadline of May 13, 2024 in Anavex Life Sciences Lawsuit - AVXL • PR Newswire (US) • 04/16/2024 09:45:00 AM
North Bay Resources Commences Operations at Bishop Gold Mill, Inyo County, California; Engages Sabean Group Management Consulting • NBRI • Sep 25, 2024 9:15 AM
CEO David B. Dorwart Anticipates a Bright Future at Good Gaming Inc. Through His Most Recent Shareholder Update • GMER • Sep 25, 2024 8:30 AM
Cannabix Technologies and Omega Laboratories Inc. Advance Marijuana Breathalyzer Technology - Dr. Bruce Goldberger to Present at Society of Forensic Toxicologists Conference • BLOZF • Sep 24, 2024 8:50 AM
Integrated Ventures, Inc Announces Strategic Partnership For GLP-1 (Semaglutide) Procurement Through MedWell USA, LLC. • INTV • Sep 24, 2024 8:45 AM
Avant Technologies Accelerates Creation of AI-Powered Platform to Revolutionize Patient Care • AVAI • Sep 24, 2024 8:00 AM
VHAI - Vocodia Partners with Leading Political Super PACs to Revolutionize Fundraising Efforts • VHAI • Sep 19, 2024 11:48 AM