Followers | 2 |
Posts | 1540 |
Boards Moderated | 0 |
Alias Born | 05/06/2017 |

Sunday, June 11, 2023 6:09:40 AM
Source
https://cj.sina.com.cn/articles/view/7651844612/1c815e20402001gkoh?from=finance
June 9, 2023
With the rapid development of technologies such as Industry 4.0, CPS (Cyber-Physical System), blockchain, cloud computing, and big data, and the rapid development of today's network and information security, access control has become the key for enterprises to protect data and resource security. The essential. In the context of ever-increasing security requirements for network and information systems, access control plays an important role in the field of network and information security as well as interdisciplinary topics in the Internet of Things. XACML (Extensible Access Control Markup Language) is a standard widely used in the field of access control. When the defined policy set becomes large and complex, the policy evaluation time will increase significantly, and the traditional policy evaluation methods often face performance bottlenecks. Performance for large-scale systems is a challenge. In order to solve this problem, WIMI (NASDAQ: WIMI) has developed DPCEngine, which is an efficient density peak clustering algorithm for improving the performance of strategy evaluation.
It is reported that WIMI WIMI Hologram Institute proposed a policy set clustering method based on the density peak clustering algorithm, which reduces the complexity of policy evaluation by identifying the cluster structure in the policy set. The architecture and algorithm flow of WIMI DPCEngine include key steps such as data preprocessing, density peak clustering, strategy matching and evaluation.
To evaluate the performance and effectiveness of DPCEngine, a real dataset containing a large-scale complex policy set is used for experiments. This dataset contains policies from different domains, covering a variety of access control scenarios. The data set is divided into training set and test set, where the training set is used to build the model of DPCEngine, and the test set is used to evaluate its performance.
WIMI Hologram R&D staff compared DPCEngine with traditional strategy evaluation methods, including methods based on linear search and tree structure.
Two performance metrics are evaluated:
- policy evaluation time and
- matching accuracy.
Policy evaluation time refers to the time required to evaluate an access request, while matching accuracy refers to the consistency between the matching results of DPCEngine and traditional methods.
DPCEngine has a significant performance advantage in policy evaluation time. Compared with traditional methods, DPCEngine can greatly reduce the policy evaluation time, especially when the policy set is large in scale and high in complexity. This is due to the density-peak-based clustering algorithm adopted by DPCEngine, which is able to cluster policy sets into smaller subsets, thereby reducing the search space for evaluation.
In terms of matching accuracy of WIMI's DPCEngine, the experimental results show that there is a high consistency between the matching results of DPCEngine and traditional methods. This shows that DPCEngine does not sacrifice accuracy while improving policy evaluation performance. In addition, we also conducted scalability experiments to evaluate the performance of DPCEngine under different scale policy sets. The results show that DPCEngine can effectively cope with large-scale policy sets and has good scalability. Its working process is shown in the figure.
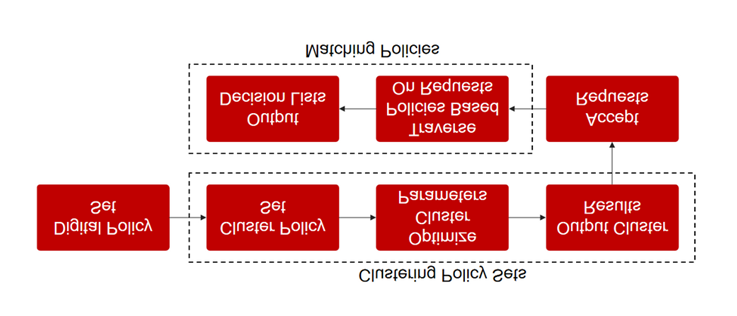
According to the data, WIMI's DPCEngine, as a strategy evaluation engine based on the density peak clustering algorithm,
has three main functions:
- preprocessing strategy set,
- cluster strategy set and
- matching strategy.
The combined use of these functions can significantly improve the performance and accuracy of policy evaluation.
Preprocessing strategy set:
Before policy evaluation, DPCEngine prepares data by preprocessing strategy sets, making it more suitable for density peak clustering. The preprocessing process includes steps such as data cleaning, feature extraction and data conversion. Ensure data accuracy and consistency by cleaning data to remove redundant, incomplete or erroneous policy information. Avoid negatively affecting the assessment results. The feature extraction process extracts key features from the policy set, such as user roles, resource types, and operation permissions, for subsequent clustering operations. Data transformation converts the strategy set into a data representation suitable for the density peak clustering algorithm, such as a vector or matrix, for cluster analysis.
Cluster policy set:
DPCEngine uses the density peak clustering algorithm to perform cluster operations on the policy set. The density peak clustering algorithm identifies the cluster structure in the policy set by evaluating the density and distance between the policies. The algorithm determines the peak point based on the density and distance between the policies, and divides the policies between the peak points into different clusters . This enables the clustering of a large and complex set of policies into smaller subsets, each cluster representing a set of policies with similar characteristics and behavioral patterns, reducing the time and complexity of policy evaluation. The result of the cluster strategy set is a group of strategy clusters with similar characteristics and behavior patterns. This cluster strategy set method can reduce the time and computational complexity of strategy evaluation, and improve the performance and efficiency of the system.
Matching strategy:
DPCEngine uses clustering results for strategy matching. When an access request arrives, DPCEngine compares and matches it with the pre-generated policy cluster. By searching for the most similar policy in each cluster, DPCEngine can quickly determine the policy set that matches the access request. This clustering-based matching method can significantly speed up policy matching and provide accurate matching results. In addition, DPCEngine can also combine other access control technologies and rule engines to further optimize the policy matching process to ensure system security and compliance.
Through the comprehensive use of preprocessing policy sets, cluster policy sets and matching policies, DPCEngine can provide enterprises with efficient and accurate policy evaluation.
In addition, WIMI's (NASDAQ: WIMI) DPCEngine's preprocessing policy set, cluster policy set and matching policy functions cooperate with each other to provide enterprises with an efficient, accurate and scalable policy evaluation performance. By utilizing the density peak clustering algorithm and the clustering structure of policy sets, DPCEngine can achieve fast policy matching in the case of large-scale complex policy sets. This cluster-based approach reduces the time and computational complexity of policy evaluation and improves the performance and efficiency of the system.
The three main functions of DPCEngine have broad application prospects in enterprises.
- First, the preprocessing policy set function can help enterprises process and prepare huge policy data to ensure data quality and consistency. This is crucial for cleaning and transforming the data prior to policy evaluation to improve the accuracy of subsequent clustering and matching.
- Second, the Cluster Policy Set feature enables enterprises to divide large and complex policy sets into relatively smaller policy clusters with similar characteristics. This cluster operation reduces the size and complexity of policy evaluation and improves the performance and efficiency of the system. By grouping similar policies together, enterprises can match access requests more quickly and implement fine-grained management and control over policies.
- Finally, the Match Policy feature allows organizations to compare and match access requests against pre-generated policy clusters. This clustering-based matching method can quickly locate the policy set that matches the access request, improving the speed and accuracy of policy matching. At the same time, DPCEngine can be used in combination with other access control technologies and rule engines to further optimize policy matching results and ensure system security and compliance.
Currently, the preprocessing policy set, cluster policy set and matching policy functions of WIMI DPCEngine enable enterprises to efficiently evaluate access control policies. This policy evaluation engine based on the density peak clustering algorithm has broad application prospects in various industries and fields, and can help enterprises build a robust security protection system and cope with growing security challenges. With the continuous development and improvement of technology, DPCEngine will further improve the performance and accuracy of policy evaluation, and provide reliable support for enterprises to ensure the security of data and resources.
Recent WIMI News
- WiMi is Working on the Blockchain Collaborative Hybrid Consensus Algorithm to Optimize Blockchain Performance and Security • PR Newswire (US) • 05/02/2024 03:40:00 PM
- WiMi Hologram Cloud Announced the B-TEC Technology to Enhance Information Security • PR Newswire (US) • 04/23/2024 03:20:00 PM
- WiMi Announced GradingShard Blockchain Sharding Technology • PR Newswire (US) • 04/22/2024 02:30:00 PM
- WiMi Announced an Efficient Connectivity Solution Based on Blockchain Lightweight Architecture 5G IoT • PR Newswire (US) • 04/18/2024 03:00:00 PM
- WiMi Developed a Hybrid Machine Learning Model Based on VMD and SVR to Lead Bitcoin Price Prediction • PR Newswire (US) • 04/08/2024 12:30:00 PM
- WiMi Developed a Lightweight Software Architecture for Payment Systems on a Blockchain Multiplexing Platform • PR Newswire (US) • 03/25/2024 02:30:00 PM
- WiMi Developed a Lightweight Multi-sectoral Data Sharing System Based on Consortium Blockchains • PR Newswire (US) • 03/21/2024 03:00:00 PM
- WiMi Announced a Bitcoin TrustBlock Validator Ensuring Secure and Reliable Transactions • PR Newswire (US) • 03/20/2024 03:40:00 PM
- WiMi Developed a Lightweight Multi-sectoral Data Sharing System Based on Consortium Blockchains • PR Newswire (US) • 03/11/2024 12:30:00 PM
- WiMi Is Researching Blockchain Optimized Data Storage Model Based on Extreme Learning Machine (ELM) Sharding • PR Newswire (US) • 03/08/2024 03:50:00 PM
- WiMi is Researching Federated Blockchain Technology to Build a Decentralized File Storage and Sharing System • PR Newswire (US) • 03/04/2024 01:30:00 PM
- WiMi Developed a New Cryptocurrency Payment System Model Based on Polygon Blockchain • PR Newswire (US) • 02/28/2024 01:30:00 PM
- WiMi Developed Efficient Prediction Models for Cryptocurrency Markets Based on Machine Learning • PR Newswire (US) • 02/27/2024 01:00:00 PM
- WiMi Developed a Blockchain-Based Data Security Model for Large Private Network Transactions • PR Newswire (US) • 02/26/2024 03:30:00 PM
- WiMi Announced MHConsensus Blockchain Algorithm Achieving Efficient Data Management • PR Newswire (US) • 02/16/2024 01:30:00 PM
- WiMi Developed K-Means Algorithm-Based Technology to Improve Security and User Trust in Bitcoin Trading Platforms • PR Newswire (US) • 02/15/2024 02:30:00 PM
- WiMi Announced Weighted PBFT to Improve Blockchain Cross-Chain Consensus Algorithm and Enhance Security and Stability of Blockchain System • PR Newswire (US) • 02/12/2024 04:30:00 PM
- WiMi Announced Multi-View Representation Learning Algorithm for Data Stream Clustering • GlobeNewswire Inc. • 02/05/2024 01:00:00 PM
- WiMi Optimized Cloud Task Scheduling in Cloud Computing Using Group Intelligence Algorithms • GlobeNewswire Inc. • 02/01/2024 01:00:00 PM
- WiMi Developed Holographic Eye-Tracking Focusing System • GlobeNewswire Inc. • 01/29/2024 01:00:00 PM
- WiMi Developed An AI-driven Real-time Spatial Interaction Perception System for Interior Design • GlobeNewswire Inc. • 01/26/2024 01:00:00 PM
- WiMi Developed Interactive System Based on V-BCI Technology Incorporated into Holographic Glasses • GlobeNewswire Inc. • 01/24/2024 01:00:00 PM
- WiMi Developed an LSTM-based Data Analysis System • GlobeNewswire Inc. • 01/22/2024 01:00:00 PM
- WiMi Developed a Trimmed K-Means Algorithm to Detect Crypto Wallet Fraud on the Bitcoin Network • GlobeNewswire Inc. • 01/18/2024 01:00:00 PM
- WiMi is Working on a New Mixed Byzantine Fault Tolerant (MBFT) Algorithm to Enhance Blockchain Fault Tolerance • GlobeNewswire Inc. • 01/16/2024 01:00:00 PM
NanoViricides Reports that the Phase I NV-387 Clinical Trial is Completed Successfully and Data Lock is Expected Soon • NNVC • May 2, 2024 10:07 AM
ILUS Files Form 10-K and Provides Shareholder Update • ILUS • May 2, 2024 8:52 AM
Avant Technologies Names New CEO Following Acquisition of Healthcare Technology and Data Integration Firm • AVAI • May 2, 2024 8:00 AM
Bantec Engaged in a Letter of Intent to Acquire a Small New Jersey Based Manufacturing Company • BANT • May 1, 2024 10:00 AM
Cannabix Technologies to Deliver Breath Logix Alcohol Screening Device to Australia • BLO • Apr 30, 2024 8:53 AM
Hydromer, Inc. Reports Preliminary Unaudited Financial Results for First Quarter 2024 • HYDI • Apr 29, 2024 9:10 AM