Followers | 2 |
Posts | 1540 |
Boards Moderated | 0 |
Alias Born | 05/06/2017 |

Saturday, May 13, 2023 7:24:36 AM
Source
https://finance.sina.com.cn/tech/roll/2023-05-12/doc-imytperx0170251.shtml
May 12, 2023
The autonomous vehicle (AV) industry is growing rapidly due to improvements in artificial intelligence (AI) technology based on sensing devices. So far, self-driving cars from as many as dozens of companies are driving on real roads for safety and performance testing. Some of them have driven tens of millions of miles, and even billions of miles in simulation. These car markets contain a large number of data sets, including all sensor data and vehicle driving information, which can be used for deep learning and provided for autonomous driving learning. These datasets include not only the vehicle's position, velocity, acceleration, but also image information obtained from cameras, light detection and ranging (LiDAR) sensors, and GPS. The information is very detailed, including not only the own vehicle, but also the information of the surrounding environment of the vehicle, which is very useful for analyzing autonomous driving problems. However, this data is so large that it takes up a lot of storage space and is difficult to analyze. Therefore, there are difficulties in testing various environments.
Based on this, WIMI (NASDAQ: WIMI) has developed a log analysis framework for autonomous vehicle test drives. Based on a formally-specified logging architecture, the framework can organize logs hierarchically, find out the prior relationships between them, and detect algorithmic or implementation errors. Vehicle logs are an essential source for detecting and analyzing events during driving. However, a set of dumped logs is usually mixed and fragmented because they are generated simultaneously by many modules such as sensors, actuators, and programs. This makes it difficult to analyze them for potential errors that may occur due to the complex chain reaction between these modules. In order to solve this problem, WIMI Hologram proposes a logging architecture based on formal specifications, and organizes the logs hierarchically to find out the prior relationship between them. Algorithmic or implementation errors are detected by examining posterior relationships, while metamorphic tests are employed to quantitatively verify formal specifications to uncover potential conflicts that may exist.
According to the information, the system is a log analysis framework for self-driving car test drives, designed to help discover potential errors that may be caused by complex chain reactions between different modules. A major component of the framework is a formal specification-based logging architecture that organizes logs hierarchically to find out their relationships. By examining these relationships, algorithmic or implementation errors can be detected.
However, testing under certain parameters does not necessarily trigger a potential conflict on the relationship. To remedy this shortcoming, the framework employs metamorphic testing to quantitatively verify the formal specification. This can help locate relationship conflicts and help improve the system. In order to verify the effectiveness of the system, the researchers constructed two experimental environments. One experimental environment is based on simulated driving tests, and the other experimental environment is based on driving log analysis techniques of actual vehicles. Both test environments used the implemented autonomous driving system to evaluate the performance and feasibility of the framework.
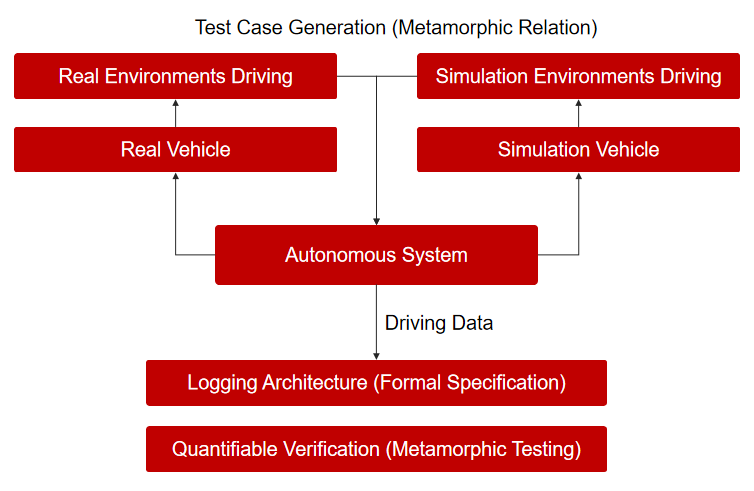
At present, the log analysis system of WIMI (NASDAQ:WIMI) self-driving car test drive is a logging framework based on formal specifications, which aims to detect and analyze the basic source of events during driving through the analysis of logs.
The technical logic of the system mainly includes the following steps:
Data collection and preprocessing:
First, the system needs to collect data from various sensors of the self-driving car. These data include the vehicle's position, velocity, attitude, acceleration, sensor data, etc. Since vehicle logs are usually mixed and fragmented since they are generated simultaneously by many modules (such as sensors, actuators, and programs), preprocessing is required for the next step of analysis.
Hierarchical organization of logs:
The system organizes the collected data hierarchically according to formal specifications. This specification includes the formal description of vehicle and environmental parameters, traffic regulations, control algorithms, and the formal definition of the execution process. Through this hierarchical organization, a priori relationship between data can be found, and then algorithm or implementation errors can be detected in subsequent analysis.
Posterior relation detection:
The system detects algorithmic or implementation errors by examining the posterior relation. By analyzing hierarchically organized logs, the system can find a posteriori relationships and detect potential errors by comparing expected and actual outcomes. If there are bugs, the bugs need to be fixed and retested and validated.
Metamorphic tests:
tests performed in the case of certain parameters (so-called oracle tests) do not necessarily trigger potential conflicts on relationships. In systems, this is remedied by employing metamorphic tests to quantitatively verify the formal specification. Variation testing is a systematic approach to varying input data to test the behavior of a system under different conditions. With this approach, the system can more fully verify its formal specification and discover potential errors and flaws.
Application example:
Finally, the market application of the system is mainly for test driving and testing of autonomous vehicles. By using the system, developers can gain a more complete understanding of how autonomous vehicles behave under different conditions and detect potential errors and flaws. This can greatly improve the safety and performance of self-driving cars and promote the development of self-driving car technology.
It can be said that WIMI Hologram provides a novel method to analyze the logs generated during the test drive of self-driving cars and help to find possible errors. It uses advanced logging techniques and testing methods to provide useful tools and techniques for those developing self-driving cars.
For the large amount of data generated when self-driving cars are tested in a real road environment, it needs to be coordinated and analyzed through a certain technical framework. Among them, the key technologies include data acquisition, data storage, data preprocessing, data analysis, etc. First of all, data acquisition requires the acquisition of vehicle driving data through a variety of sensors, including visual sensors, lidar, millimeter-wave radar, inertial navigation systems, etc., to obtain information about the surrounding environment of the vehicle and the state of the vehicle. Second, data storage requires efficient storage and management of acquired data. Distributed file systems and database technologies are usually used to ensure high reliability and scalability of data. At the same time, the data needs to be stored in a certain format to facilitate subsequent data preprocessing and analysis.
Data preprocessing includes data cleaning, denoising and feature extraction. In autonomous vehicle testing, the acquired data is noisy because sensors may be disturbed by various factors. Therefore, it is necessary to denoise the data to ensure the accuracy of subsequent data analysis and visualization. In addition, through feature extraction, key information in the data can be extracted for subsequent data analysis. Data analysis requires the analysis of data through techniques such as machine learning and deep learning. Among them, analysis methods based on statistics, methods based on machine learning and methods based on deep learning are included. Through data analysis, evaluation results on the performance and safety of self-driving cars can be obtained, as well as optimization suggestions. Through the realization of the above technical logic, the test data of self-driving cars can be coordinated and analyzed in an all-round way to provide support for the performance and safety of self-driving cars.
Self-driving cars are an important part of future transportation, and their market prospects are huge. According to market research firm forecasts, by 2025, the global autonomous vehicle market will exceed $200 billion. At present, self-driving car technology has received extensive attention from many automakers and technology companies, including Tesla, Google, Uber, etc. These companies are constantly advancing their self-driving car technology and investing heavily in research and development. WIMI (NASDAQ: WIMI) will also continue to promote the development of software and data analysis technology related to self-driving cars, making contributions to the development of the industry. greater contribution.
Recent WIMI News
- WiMi Developed a Swin-Transformer and Hash-based On-Chain Copyright Detection Technology • PR Newswire (US) • 05/06/2024 02:50:00 PM
- WiMi is Working on the Blockchain Collaborative Hybrid Consensus Algorithm to Optimize Blockchain Performance and Security • PR Newswire (US) • 05/02/2024 03:40:00 PM
- WiMi Hologram Cloud Announced the B-TEC Technology to Enhance Information Security • PR Newswire (US) • 04/23/2024 03:20:00 PM
- WiMi Announced GradingShard Blockchain Sharding Technology • PR Newswire (US) • 04/22/2024 02:30:00 PM
- WiMi Announced an Efficient Connectivity Solution Based on Blockchain Lightweight Architecture 5G IoT • PR Newswire (US) • 04/18/2024 03:00:00 PM
- WiMi Developed a Hybrid Machine Learning Model Based on VMD and SVR to Lead Bitcoin Price Prediction • PR Newswire (US) • 04/08/2024 12:30:00 PM
- WiMi Developed a Lightweight Software Architecture for Payment Systems on a Blockchain Multiplexing Platform • PR Newswire (US) • 03/25/2024 02:30:00 PM
- WiMi Developed a Lightweight Multi-sectoral Data Sharing System Based on Consortium Blockchains • PR Newswire (US) • 03/21/2024 03:00:00 PM
- WiMi Announced a Bitcoin TrustBlock Validator Ensuring Secure and Reliable Transactions • PR Newswire (US) • 03/20/2024 03:40:00 PM
- WiMi Developed a Lightweight Multi-sectoral Data Sharing System Based on Consortium Blockchains • PR Newswire (US) • 03/11/2024 12:30:00 PM
- WiMi Is Researching Blockchain Optimized Data Storage Model Based on Extreme Learning Machine (ELM) Sharding • PR Newswire (US) • 03/08/2024 03:50:00 PM
- WiMi is Researching Federated Blockchain Technology to Build a Decentralized File Storage and Sharing System • PR Newswire (US) • 03/04/2024 01:30:00 PM
- WiMi Developed a New Cryptocurrency Payment System Model Based on Polygon Blockchain • PR Newswire (US) • 02/28/2024 01:30:00 PM
- WiMi Developed Efficient Prediction Models for Cryptocurrency Markets Based on Machine Learning • PR Newswire (US) • 02/27/2024 01:00:00 PM
- WiMi Developed a Blockchain-Based Data Security Model for Large Private Network Transactions • PR Newswire (US) • 02/26/2024 03:30:00 PM
- WiMi Announced MHConsensus Blockchain Algorithm Achieving Efficient Data Management • PR Newswire (US) • 02/16/2024 01:30:00 PM
- WiMi Developed K-Means Algorithm-Based Technology to Improve Security and User Trust in Bitcoin Trading Platforms • PR Newswire (US) • 02/15/2024 02:30:00 PM
- WiMi Announced Weighted PBFT to Improve Blockchain Cross-Chain Consensus Algorithm and Enhance Security and Stability of Blockchain System • PR Newswire (US) • 02/12/2024 04:30:00 PM
- WiMi Announced Multi-View Representation Learning Algorithm for Data Stream Clustering • GlobeNewswire Inc. • 02/05/2024 01:00:00 PM
- WiMi Optimized Cloud Task Scheduling in Cloud Computing Using Group Intelligence Algorithms • GlobeNewswire Inc. • 02/01/2024 01:00:00 PM
- WiMi Developed Holographic Eye-Tracking Focusing System • GlobeNewswire Inc. • 01/29/2024 01:00:00 PM
- WiMi Developed An AI-driven Real-time Spatial Interaction Perception System for Interior Design • GlobeNewswire Inc. • 01/26/2024 01:00:00 PM
- WiMi Developed Interactive System Based on V-BCI Technology Incorporated into Holographic Glasses • GlobeNewswire Inc. • 01/24/2024 01:00:00 PM
- WiMi Developed an LSTM-based Data Analysis System • GlobeNewswire Inc. • 01/22/2024 01:00:00 PM
- WiMi Developed a Trimmed K-Means Algorithm to Detect Crypto Wallet Fraud on the Bitcoin Network • GlobeNewswire Inc. • 01/18/2024 01:00:00 PM
FEATURED POET Announces Design Win and Collaboration with Foxconn Interconnect Technology for High-speed AI Systems • May 14, 2024 10:09 AM
FEATURED Element79 Gold Corp Reports Exceptionally High-Grade Results from Lucero • May 14, 2024 7:00 AM
VAYK Added New Manager for Expansion into $64 Billion Domestic Short-term Rental Market • VAYK • May 14, 2024 9:00 AM
Avant Technologies Equipping AI-Managed Data Center with High Performance Computing Systems • AVAI • May 10, 2024 8:00 AM
VAYK Discloses Strategic Conversation on Potential Acquisition of $4 Million Home Service Business • VAYK • May 9, 2024 9:00 AM
Bantec's Howco Awarded $4.19 Million Dollar U.S. Department of Defense Contract • BANT • May 8, 2024 10:00 AM