Followers | 2 |
Posts | 1540 |
Boards Moderated | 0 |
Alias Born | 05/06/2017 |

Monday, May 01, 2023 8:16:58 PM
Source
https://finance.sina.com.cn/tech/roll/2023-04-27/doc-imyrvfyt3148265.shtml
April 27, 2023
In recent years, self-driving car technology has made great progress with the development of technology, but how to ensure the safety of self-driving cars in various complex scenarios is still a very challenging problem. Traditional verification methods based on manual and drive tests have great limitations in terms of time and cost. The occurrence of traffic accidents, especially in extreme situations, is a key bottleneck hindering the development and deployment of autonomous vehicles. Since safety-critical events are rare, the economic and time costs required to verify their safety in natural driving environments are prohibitively high. Daunting.
According to reports, WIMI (NASDAQ: WIMI) is developing an intensive reinforcement learning technology for safety verification of self-driving cars. Fast validation and training in the emulator.
According to the data, WIMI Hologram's simulator based on machine-intensive reinforcement learning is a model-based machine-intensive reinforcement learning technology for safety verification of autonomous vehicles. The technology trains an agent in a simulator and puts it through a dense reinforcement learning algorithm for validation in a natural driving environment.
Dense reinforcement learning (DRL) is a machine learning technique that enables machine intelligence agents to learn and make optimal decisions from their interactions with the environment. In the field of self-driving cars, dense reinforcement learning is used to develop safety verification systems to ensure that self-driving cars can drive correctly in various situations. When using machine-dense reinforcement learning (DRL) for self-driving car safety verification, it is usually divided into two phases:
training and verification.
During the training phase, the DRL agent interacts with the environment and learns from it. During the validation phase, the DRL agent is tested in a simulator or in the real world to see if it can drive correctly and make optimal decisions. The safety verification of autonomous vehicles is a very complex and time-consuming process, as its safety needs to be verified in various road and traffic scenarios. Dense Simulator Reinforcement Learning is a technique for safety verification of self-driving cars using a simulator, which can greatly reduce the time and cost of verification.
It is reported that the intensive reinforcement learning technology of WIMI (NASDAQ: WIMI) adopts the model-based reinforcement learning (Model-Based Reinforcement Learning) method, which combines the idea of ??model predictive control. Specifically, we built a model in the simulator to predict the agent's actions and possible consequences in the current environment, and calculate various possibilities. We then use reinforcement learning algorithms to optimize the agent's policy to best meet goals such as safety and efficiency.
Compared with traditional reinforcement learning methods, machine-dense reinforcement learning-based simulators have higher efficiency and stability. By using the model, we can quickly generate a large amount of training data in the simulator, and can better control the environment and state in the simulator, so as to better approximate the real world situation. In addition, the method of Multi-Agent Reinforcement Learning is adopted to allow different agents to cooperate with each other in the simulator, so as to better adapt to complex autonomous vehicle scenarios. In training, we also use technical means such as Experience Replay (ER), Priority Experience Replay (PER), Dynamic Time Discount (DTD), etc. to improve the efficiency and stability of training.
At present, WIMI (NASDAQ: WIMI) is based on a machine-intensive reinforcement learning simulator. Through the process of defining the agent's goal and environment, establishing an agent model, training the agent, and using the dense simulator reinforcement learning technology for training and verification, the simulation Large-scale testing and verification in the device greatly reduces the cost and time of testing and verification in the real world, and improves the development efficiency and quality of autonomous vehicles.
The technical implementation process is as follows:
Construction of driving scene simulator:
firstly, it is necessary to build a driving scene simulator, which can simulate various road and traffic scenes. A simulator needs to include elements such as vehicles, pedestrians, roads, traffic lights, and the physics and behavioral rules associated with them.
Define the agent's goals and environment:
It is necessary to clarify the agent's goals and the environment to face. For example, an agent's goal might be to reach a destination in the shortest possible time while minimizing accidents. The environment includes roads, traffic lights, other vehicles, pedestrians, etc.
Establish an agent model:
establish an agent model, including input, output, network structure, etc. For example, the input might include information such as the agent's current speed, location, and the location and speed of surrounding vehicles; the output might be the next action the agent should take, such as accelerating, decelerating, turning, etc. When building an agent model, the working environment of the agent in the real world needs to be considered, and relevant traffic rules and safety requirements need to be followed.
Training Agents:
Agents require extensive training and experimentation in an emulator. An algorithm based on reinforcement learning can be used to improve the performance of the agent through continuous trial and error and learning. The agent needs to constantly explore new strategies and adjust its behavior according to the reward signal to maximize the long-term cumulative reward.
Dense Reinforcement Learning Simulator:
Dense reinforcement learning simulator refers to accelerating the learning and validation process of an agent by conducting a large number of training and experiments in a simulator in a short period of time. Specifically, some technologies can be used to speed up the operation of the simulator, such as parallel computing, distributed computing, and so on. At the same time, some technologies can also be used to automatically generate various road and traffic scenarios to improve the efficiency of training and verification.
Validate the agent:
After training in the simulator, the agent needs to be deployed in the real world for validation. During the verification process, some techniques can be used to speed up the verification of the agent, such as gradually relaxing the environmental restrictions, gradually increasing the complexity of the scene, artificially introducing interference, etc. If the agent performs well in the real world, the complexity of the scenario in the simulator can be further increased to verify the safety of the agent with stricter standards.
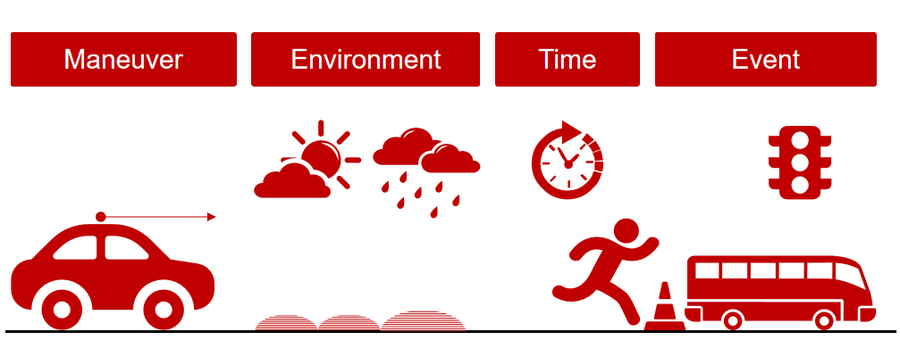
Typically, the development and deployment of autonomous vehicles requires extensive testing and validation that needs to be done in the real world, which is time- and cost-prohibitive. Through the WIMI holographic intensive reinforcement learning simulator technology, large-scale testing and verification can be carried out in the simulator, which greatly reduces the cost and time of testing and verification in the real world, and improves the development efficiency and efficiency of autonomous vehicles. quality. Secondly, self-driving cars face many complex scenarios and environments in the real world, such as weather changes, road conditions, behaviors of other vehicles and pedestrians, etc.
These complex scenarios and environments are difficult to reproduce and verify in the real world. Through the intensive simulator reinforcement learning technology in the simulator, these complex scenarios and environments can be simulated, and the performance and safety of the self-driving car can be trained and verified in the simulator, so that it can better cope with the challenges in the real world. In the verification process, the verification process can also be accelerated by gradually relaxing the environmental restrictions, gradually increasing the complexity of the scene, and artificially introducing interference. This technique could greatly reduce the time and cost of safety verification while improving the safety and reliability of autonomous vehicles.
In short, WIMI (NASDAQ: WIMI) adopts intensive reinforcement learning simulator technology, which can help the autonomous driving industry to verify and train the safety of autonomous vehicles more quickly, efficiently and accurately. This will bring more reliable and safer self-driving car products to users, and will also accelerate the development and popularization of self-driving car technology. Intensive reinforcement learning technology provides a brand-new solution for the development and verification of self-driving cars, laying a solid foundation for advancing the development and application of self-driving cars. It is believed that self-driving car safety verification technology based on intensive reinforcement learning will be An important trend and direction in the future.
Recent WIMI News
- WiMi Announced a Blockchain Consensus Mechanism Based on Improved Distributed Consistency and Hash Entropy • PR Newswire (US) • 07/01/2024 02:30:00 PM
- WiMi Announced a Intelligent Interconnected Decision-making System Based on Blockchain Technology • PR Newswire (US) • 06/12/2024 02:30:00 PM
- WIMI Announced to Jointly Establish a Micro-Consciousness Quantum Research Center With MicroAlgo • PR Newswire (US) • 06/04/2024 08:06:00 AM
- WiMi Developed BlockChain Guardian Technology to Enable IIOT Cybersecurity • PR Newswire (US) • 06/03/2024 02:50:00 PM
- WiMi Announced the Internet of Things (IoT) Data Perception Based on Blockchain Technology • PR Newswire (US) • 05/24/2024 03:10:00 PM
- WiMi Announced a Blockchain Data Encryption Technology Based on Machine Learning and Fully Homomorphic Encryption Algorithm • PR Newswire (US) • 05/22/2024 04:54:00 PM
- WiMi Announced PoLe Blockchain Consensus Mechanism to Enable Efficient Training of Neural Networks • PR Newswire (US) • 05/17/2024 03:58:00 PM
- WiMi Announced the Innovative Application of RAFT Consensus Algorithm in Blockchain to Build a Secure Data Management Model • PR Newswire (US) • 05/15/2024 02:20:00 PM
- WiMi Developed a Swin-Transformer and Hash-based On-Chain Copyright Detection Technology • PR Newswire (US) • 05/06/2024 02:50:00 PM
- WiMi is Working on the Blockchain Collaborative Hybrid Consensus Algorithm to Optimize Blockchain Performance and Security • PR Newswire (US) • 05/02/2024 03:40:00 PM
- WiMi Hologram Cloud Announced the B-TEC Technology to Enhance Information Security • PR Newswire (US) • 04/23/2024 03:20:00 PM
- WiMi Announced GradingShard Blockchain Sharding Technology • PR Newswire (US) • 04/22/2024 02:30:00 PM
- WiMi Announced an Efficient Connectivity Solution Based on Blockchain Lightweight Architecture 5G IoT • PR Newswire (US) • 04/18/2024 03:00:00 PM
- WiMi Developed a Hybrid Machine Learning Model Based on VMD and SVR to Lead Bitcoin Price Prediction • PR Newswire (US) • 04/08/2024 12:30:00 PM
- WiMi Developed a Lightweight Software Architecture for Payment Systems on a Blockchain Multiplexing Platform • PR Newswire (US) • 03/25/2024 02:30:00 PM
- WiMi Developed a Lightweight Multi-sectoral Data Sharing System Based on Consortium Blockchains • PR Newswire (US) • 03/21/2024 03:00:00 PM
- WiMi Announced a Bitcoin TrustBlock Validator Ensuring Secure and Reliable Transactions • PR Newswire (US) • 03/20/2024 03:40:00 PM
- WiMi Developed a Lightweight Multi-sectoral Data Sharing System Based on Consortium Blockchains • PR Newswire (US) • 03/11/2024 12:30:00 PM
- WiMi Is Researching Blockchain Optimized Data Storage Model Based on Extreme Learning Machine (ELM) Sharding • PR Newswire (US) • 03/08/2024 03:50:00 PM
- WiMi is Researching Federated Blockchain Technology to Build a Decentralized File Storage and Sharing System • PR Newswire (US) • 03/04/2024 01:30:00 PM
- WiMi Developed a New Cryptocurrency Payment System Model Based on Polygon Blockchain • PR Newswire (US) • 02/28/2024 01:30:00 PM
- WiMi Developed Efficient Prediction Models for Cryptocurrency Markets Based on Machine Learning • PR Newswire (US) • 02/27/2024 01:00:00 PM
- WiMi Developed a Blockchain-Based Data Security Model for Large Private Network Transactions • PR Newswire (US) • 02/26/2024 03:30:00 PM
- WiMi Announced MHConsensus Blockchain Algorithm Achieving Efficient Data Management • PR Newswire (US) • 02/16/2024 01:30:00 PM
- WiMi Developed K-Means Algorithm-Based Technology to Improve Security and User Trust in Bitcoin Trading Platforms • PR Newswire (US) • 02/15/2024 02:30:00 PM
Freedom Holdings Corporate Update; Announces Management Has Signed Letter of Intent • FHLD • Jul 3, 2024 9:00 AM
EWRC's 21 Moves Gaming Studios Moves to SONY Pictures Studios and Green Lights Development of a Third Upcoming Game • EWRC • Jul 2, 2024 8:00 AM
BNCM and DELEX Healthcare Group Announce Strategic Merger to Drive Expansion and Growth • BNCM • Jul 2, 2024 7:19 AM
NUBURU Announces Upcoming TV Interview Featuring CEO Brian Knaley on Fox Business, Bloomberg TV, and Newsmax TV as Sponsored Programming • BURU • Jul 1, 2024 1:57 PM
Mass Megawatts Announces $220,500 Debt Cancellation Agreement to Improve Financing and Sales of a New Product to be Announced on July 11 • MMMW • Jun 28, 2024 7:30 AM
VAYK Exited Caribbean Investments for $320,000 Profit • VAYK • Jun 27, 2024 9:00 AM