Followers | 2 |
Posts | 1540 |
Boards Moderated | 0 |
Alias Born | 05/06/2017 |

Sunday, February 05, 2023 3:00:25 AM
Source
https://cj.sina.com.cn/articles/view/1704103183/65928d0f020032xz2?from=finance
February 03, 2023 11:20 Financial World Website
The amount of Internet data is growing explosively, the impact of data on social production and life is increasing, and the application of data mining technology is becoming more and more extensive. The data mining process is a repeated human-computer interaction process, which includes defining problems, establishing data mining databases, analyzing data, preparing data, establishing models, evaluating models, and so on. The main data mining algorithms include association analysis, sequential pattern mining, classification algorithms and clustering algorithms.
It is understood that WIMI Hologram (NASDAQ: WIMI) has developed a data mining clustering optimization algorithm system based on artificial neural networks. In cluster analysis, the data is divided according to certain rules. After the data is divided into categories, the similarity between the categories is small and the similarity within the category is large. The results of data analysis can not only reveal the internal connections and differences between data, but also provide an important basis for further data analysis and knowledge discovery.
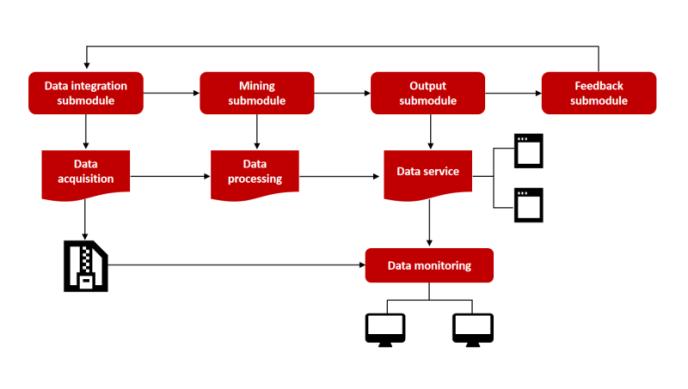
WIMI's development of data mining clustering optimization algorithms based on artificial neural networks includes several methods:
(1) Partition method:
This method can find spherical mutually exclusive clusters, and the center of the cluster is represented by the mean or center point. This algorithm is suitable for clustering problems with a fixed number of clusters and small data sets. Through the random search strategy, large-scale data clustering has high efficiency and good scalability. Partition clustering algorithms are usually easy to parallelize and have been very active on big data processing platforms in recent years.
(2) Hierarchical method:
This method is based on the idea of ??hierarchical decomposition clustering, which performs hierarchical decomposition according to the similarity between data points, and generates a nested clustering tree with a hierarchical structure. The bottom-up hierarchical decomposition corresponds to the agglomerative method, and the top-down hierarchical decomposition corresponds to the split method.
(3) Density-based approach:
This algorithm can find clusters with different regular shapes without forcing the shape of the clusters to change. It is suitable for clusters with irregular numbers and random shapes, and has the advantage of reducing or even eliminating noise. It divides the area with sufficient density into clusters, and finds clusters of arbitrary shape in the noisy spatial database. According to the local density of the sampling points, the cluster is defined as the largest set of points with connection density.
(4) Grid-based method:
This algorithm clusters the quantized grid space, which is fast and has strong computing power. Divide the space into grids and perform analysis on the data on the grids.
(5) Model clustering:
Model clustering algorithms assume that data are mixed according to a specific probability distribution that strives to find the best fit between the data and a given model.
In this era of massive data, data mining is extremely important, its application is becoming more and more extensive, and its importance is becoming more and more obvious. As long as the enterprise has a data warehouse or database with analytical value and needs, it can carry out purposeful data mining and obtain valuable data.
While the clustering optimization algorithm can deal with data with multi-dimensional and irrelevant characteristics, the choice of clustering method directly determines the quality of data mining. In order to improve the quality of clustering, people continue to explore better clustering analysis methods.
The artificial neural network-based data mining clustering optimization algorithm developed by WIMI can automatically merge the clustering results with smaller granularity according to the preset warning value, thus effectively preventing unreasonable clustering caused by too many specified clusters. Class results occur. Artificial neural network has highly nonlinear learning ability and fault-tolerant ability to noisy data, and it has a strong ability to extract rule knowledge. Using artificial neural network model to process data and mine knowledge has very obvious advantages.
Recent WIMI News
- WiMi Developed a Blockchain-Based Integrated Architecture for Security Maintenance in Cloud Computing • PR Newswire (US) • 09/19/2024 01:30:00 PM
- WiMi Developed a Blockchain Empowered Asynchronous Federated Learning for Optimizing Model Training • PR Newswire (US) • 09/09/2024 02:30:00 PM
- WiMi Hologram Cloud Announced an Identity Management Model Based on Blockchain • PR Newswire (US) • 08/23/2024 03:00:00 PM
- Form F-3 - Registration statement by foreign private issuers • Edgar (US Regulatory) • 08/09/2024 10:12:37 AM
- WiMi Developed a Payment Channel Rebalancing Model for Layer-2 Blockchain • PR Newswire (US) • 08/08/2024 02:40:00 PM
- WiMi Announced a Secure and Trusted Collaborative Learning Based on Blockchain for IoT • PR Newswire (US) • 08/06/2024 01:00:00 PM
- WiMi Announced a Blockchain-based Framework for Secure Data Sharing in Smart Cities • PR Newswire (US) • 07/29/2024 01:00:00 PM
- WiMi Built an Advanced Data Structure Architecture Using Homomorphic Encryption and Federated Learning • PR Newswire (US) • 07/26/2024 01:00:00 PM
- WiMi Developed a Blockchain-Based Trust Approach for Cloud Computing to Address Security in Services • PR Newswire (US) • 07/19/2024 03:00:00 PM
- WiMi Announced a Genetic Algorithm-based Consensus Algorithm for Blockchain • PR Newswire (US) • 07/15/2024 01:30:00 PM
- WiMi Announced a Blockchain Consensus Mechanism Based on Improved Distributed Consistency and Hash Entropy • PR Newswire (US) • 07/01/2024 02:30:00 PM
- WiMi Announced a Intelligent Interconnected Decision-making System Based on Blockchain Technology • PR Newswire (US) • 06/12/2024 02:30:00 PM
- WIMI Announced to Jointly Establish a Micro-Consciousness Quantum Research Center With MicroAlgo • PR Newswire (US) • 06/04/2024 08:06:00 AM
- WiMi Developed BlockChain Guardian Technology to Enable IIOT Cybersecurity • PR Newswire (US) • 06/03/2024 02:50:00 PM
- WiMi Announced the Internet of Things (IoT) Data Perception Based on Blockchain Technology • PR Newswire (US) • 05/24/2024 03:10:00 PM
- WiMi Announced a Blockchain Data Encryption Technology Based on Machine Learning and Fully Homomorphic Encryption Algorithm • PR Newswire (US) • 05/22/2024 04:54:00 PM
- WiMi Announced PoLe Blockchain Consensus Mechanism to Enable Efficient Training of Neural Networks • PR Newswire (US) • 05/17/2024 03:58:00 PM
- WiMi Announced the Innovative Application of RAFT Consensus Algorithm in Blockchain to Build a Secure Data Management Model • PR Newswire (US) • 05/15/2024 02:20:00 PM
- WiMi Developed a Swin-Transformer and Hash-based On-Chain Copyright Detection Technology • PR Newswire (US) • 05/06/2024 02:50:00 PM
- WiMi is Working on the Blockchain Collaborative Hybrid Consensus Algorithm to Optimize Blockchain Performance and Security • PR Newswire (US) • 05/02/2024 03:40:00 PM
- WiMi Hologram Cloud Announced the B-TEC Technology to Enhance Information Security • PR Newswire (US) • 04/23/2024 03:20:00 PM
- WiMi Announced GradingShard Blockchain Sharding Technology • PR Newswire (US) • 04/22/2024 02:30:00 PM
- WiMi Announced an Efficient Connectivity Solution Based on Blockchain Lightweight Architecture 5G IoT • PR Newswire (US) • 04/18/2024 03:00:00 PM
- WiMi Developed a Hybrid Machine Learning Model Based on VMD and SVR to Lead Bitcoin Price Prediction • PR Newswire (US) • 04/08/2024 12:30:00 PM
- WiMi Developed a Lightweight Software Architecture for Payment Systems on a Blockchain Multiplexing Platform • PR Newswire (US) • 03/25/2024 02:30:00 PM
VHAI - Vocodia Partners with Leading Political Super PACs to Revolutionize Fundraising Efforts • VHAI • Sep 19, 2024 11:48 AM
Dear Cashmere Group Holding Co. AKA Swifty Global Signs Binding Letter of Intent to be Acquired by Signing Day Sports • DRCR • Sep 19, 2024 10:26 AM
HealthLynked Launches Virtual Urgent Care Through Partnership with Lyric Health. • HLYK • Sep 19, 2024 8:00 AM
Element79 Gold Corp. Appoints Kevin Arias as Advisor to the Board of Directors, Strengthening Strategic Leadership • ELMGF • Sep 18, 2024 10:29 AM
Mawson Finland Limited Further Expands the Known Mineralized Zones at Rajapalot: Palokas step-out drills 7 metres @ 9.1 g/t gold & 706 ppm cobalt • MFL • Sep 17, 2024 9:02 AM
PickleJar Announces Integration With OptCulture to Deliver Holistic Fan Experiences at Venue Point of Sale • PKLE • Sep 17, 2024 8:00 AM