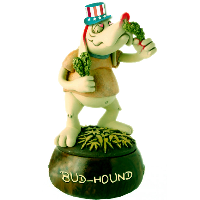
Thursday, December 18, 2008 10:16:21 PM
<<<...that maybe can fill in some of the gaps in my understanding. >>>
Introduction
Precis
Welcome to the era of Genomic and Personalized Medicine. This article will tell you what you need to know and how to be best armed to take full advantage of emerging genomic information and incorporate it into the daily practice of medicine.
Medical Practice Circa 2015
Consider this case example of how genomics might be integrated into medical practice within the next 10 years:
Jackie, a 50-year-old woman, had her genome scanned 4 years ago, in 2011, by her physician and was found to have 5 genetic variants that increase her risk for heart disease threefold; she also had 3 genetic variants that confer protection against cancer. Recommendations for her diet and medications have been made on the basis of her genetic profile for metabolizing nutrients and specific medication classes. During her last examination, she underwent a screening blood-based profile for coronary artery disease that not only showed that her atherosclerotic disease burden was high but also that her overall risk for developing an acute coronary syndrome in the next 5 years was high. When chest pain developed recently, a blood-based molecular biosignature confirmed ischemia caused by intermittent thrombosis without necrosis. Targeted anti-inflammatory and individualized dosing regimens for anticoagulant medications were prescribed and symptoms did not recur.
Background
Medicine today already contemplates the use of molecular markers to predict health risks. Glucose and cholesterol measurements, for example, are standard of care. However, whereas these are good screening tests for occult disease, they are not necessarily 'predictive' of future events. Genomic information provides the opportunity to develop and refine the prediction for risk and increase the precision of that prediction for the individual. Sir William Osler (1849-1919) recognized that "variability is the law of life, and as no two faces are the same, so no two bodies are alike, and no two individuals react alike and behave alike under the abnormal conditions which we know as disease."[1] We now have a new set of tools that can be used to understand biological and disease variability. However, health and disease management today are further complicated by a pharmaceutical industry that has developed medications using a one-size-fits-all paradigm rather than medications tailored to human variability. Thus, in medical practice today, many drugs work in fewer than 50% of the patients to whom they are prescribed. Furthermore, more than 100,000 people die annually from drug-related adverse events -- a top 10 cause of death in 1994.[2] In Britain, it was recently estimated that adverse drug reactions are responsible for 6.5% of that country's hospital admissions and for nearly $1 billion in annual costs to the National Health Service.[3] A more personalized or customized approach to medical care might be part of the solution to these healthcare woes.
What Is Personalized Medicine?
Personalized medicine is a rapidly advancing field of healthcare that is informed by each person's unique clinical, genetic (DNA-based), genomic (whole genome or its products), and environmental information.[4] The goals of personalized medicine are to take advantage of a molecular understanding of disease to optimize preventive healthcare strategies and drug therapies while people are still well or at the earliest stages of disease. Because these factors are different for every person, the nature of disease, its onset, its course, and how it might respond to drug or other interventions are as individual as the people who have them. For personalized medicine to be used by healthcare providers and their patients, these findings must be translated into precision diagnostic tests and targeted therapies. Because the overarching goal is to optimize medical care and outcomes for each individual, treatments, medication types and dosages, and/or prevention strategies may differ from person to person -- resulting in unprecedented customization of patient care.
Specific advantages that personalized medicine may offer patients and clinicians include the following:
The ability to make more informed medical decisions;
Higher probability of desired outcomes thanks to better-targeted therapies;
Reduced probability of adverse side effects;
Focus on prevention and prediction of disease rather than reaction to it;
Earlier disease intervention; and
Reduced healthcare costs.
What is Genomic Medicine?
Genomic medicine is an essential component of the broader personalized medicine concept. Simply defined, it is the use of genomic information to guide medical decision making.[5] The prospect of examining a person's entire genome (or at least a large fraction of it) to make individualized risk predictions and treatment decisions is tantalizingly within reach. Since the completion of the sequence of the human genome, genomic information has been used, albeit on a small scale, to tailor care to individual patients. The opportunity, however, is enormous: for the first time, we are in a position to characterize health and disease states by their molecular fingerprints, develop meaningful stratifiers for patient populations, elucidate mechanistic pathways based on genome-wide data, and develop new preventive, diagnostic, and therapeutic strategies that will shift the focus of care from intervention to prevention.
What Is the Human Genome and How Does it Vary From Person to Person?
The human genome is composed of approximately 25,000 genes defined among 3 billion bases or nucleotides of DNA residing on 46 chromosomes. Variation in this sequence from one individual to another can occur even at the level of a single base (termed a single nucleotide polymorphism [SNP]). Other types of variation include deletions, insertions, repetitive elements, and large chromosomal rearrangements. All of these may have medical implications, with the latter (deletions, insertions, repetitive DNA elements, and large chromosomal rearrangements) being responsible for a large number of congenital malformations and inherited single-gene diseases. The former -- the SNPs -- largely underlie complex human diseases and their contribution to these diseases has been among the most challenging roles to define. A polymorphism is a genetic variant that has 2 alleles in a population at a frequency of > 1%. SNPs are the most common form of genetic variation and there are an estimated 10,000,000 SNPs in the human genome. High-throughput technologies such as direct sequencing, various genotyping platforms, and microarrays enable rapid and accurate analyses from very small quantities of blood or tissue samples. SNPs are classified as non-synonymous if they cause an altered protein amino acid sequence and synonymous or neutral if protein amino acid sequence is unchanged. They may also fall in an intergenic region (eg, intron, promoter, untranslated region). Any of these may lead to disease caused by changes in protein structure or function as a result of amino acid changes or alternative splicing or by changes in protein quantity as a result of altered transcription factor-binding sites or other regulatory disruptions.
Genetic variation is complex at a population level, with many SNPs existing in different populations (of different ethnic origins) and at different frequencies. To simplify which SNPs should be measured in various populations, the International Hap Map Project was started in 2002 as a multicountry effort to identify, catalogue, and make publicly available the genetic diversity in humans.[6] The HapMap has identified which SNPs travel with others in blocks of the genome (a phenomenon termed linkage disequilibrium) such that the measurement of one SNP is informative for a large number of neighboring SNPs that therefore do not have to be directly measured. A complete version of the HapMap can be found at http://www.hapmap.org. The HapMap project has been important in facilitating genetic discovery -- investigators can now focus on those SNPs that are informative about a series of SNPs in a single block of the genome. This reduces the number of SNPs needed to test for genetic studies, increasing the speed of the studies and decreasing their cost.
SNPs are increasingly being used in clinical practice for the diagnosis of Mendelian disorders such as cystic fibrosis and long QT syndrome. However, there are few, if any, SNPs used in clinical decision making for complex diseases such as asthma or autism. The multigenic nature of these common diseases, the modest effect of the genetic variant(s), and the confounding effects of environmental influences have made them exceedingly difficult to validate in multiple populations. A predictive genetic test for a complex disease would likely consist of a panel of multiple SNPs combined with other genomic and clinical information.[7]
What Are the Types of Genomic Information?
Having access to the entire human sequence is a necessary but insufficient prerequisite for genomic medicine. What is equally important is having the technology at hand to reliably visualize individual genomes (as well as their derivatives, the transcriptome, proteome, and metabolome) for health and disease status (Table).
Table. The Genomic Medicine Toolbox
Dataset (-omic approach) Technology Platform or Approach
Human genome sequence (genomics) SNPs, estimated ~ 10 million
Gene expression profiles (transcriptomics) Microarrays of ~ 25,000 RNA gene transcripts
Proteome (proteomics) Protein profiles of specific protein products (~ 100,000)
Metabolome (metabolomics) Metabolic profiles (1000 to 10,000 metabolites)
SNP = single nucleotide polymorphisms
Gene Expression Variation (Transcriptomics). Gene expression profiles can also serve as important markers for disease and clinical outcomes. Gene expression profiles generated from microarrays of more than 22,500 transcripts characterize overall transcriptional activity in a particular cell type as it is affected by gene sequence variation, regulatory controls, and environmental influences. Gene expression patterns as a whole are commonly used in classification and predictive models to differentiate tumors that are similar histologically. Microarrays -- small, solid supports onto which the sequences from thousands of different genes are immobilized, or attached, at fixed locations -- are the major tool used to measure genome-wide changes in gene expression; however, older platforms such as reverse transcriptase polymerase chain reaction are routinely used for smaller gene sets. This approach has been used experimentally for classifying acute myelogenous leukemia subtypes, for distinguishing Burkitt's from diffuse large B-cell lymphoma, for predicting outcomes in breast and lung cancers, and for assessing atherosclerotic burden in human aortas.[8-14]
Although patterns of gene expression composing a molecular profile or signature that correlates with a phenotype can be observed, it is often unclear what the relationship is between the pattern and the cellular pathways or networks relevant to the disease process in question. For example, an expression profile of 16 cancer-related genes has been associated with the risk for breast cancer recurrence, but it is not yet clear how each of the genes function, whether separately or together, in the pathophysiology of breast cancer.[14] A noninvasive molecular expression profile has also been developed and validated to categorize heart transplant patients into low- and high-risk categories for cardiac rejection before clinical symptoms appear[15] Again, the pattern is predictive, but the role of the genes in allograft rejection is not yet known. Recently, genomics-based assays have been released commercially to aid in medical management of breast cancer treatment[16] and cardiac transplant.[17]
Protein Expression (Proteomics) Assays. Proteomics involves the large-scale study of the structure, quantity, and function of proteins and provides a comprehensive dataset about cellular networks, interactions, and products. Proteomics naturally followed the field of genomics to characterize the protein make-up of cells in different tissues, organisms, and disease states.[18] The proteome is estimated to contain approximately 100,000 protein entities and their post-transcriptionally modified derivatives. One of the major challenges to this young field is distinguishing protein signatures linked to a specific drug or disease from background caused by confounding responses to environment, lifestyle, or comorbidities. While still in its early days for diagnosis and prognosis, multiplex proteomic assays used to detect a profile of multiple proteins are being developed for disease management. A combined measure of C-reactive protein, B-type natriuretic peptide, and cardiac troponin I can be used for risk stratification of patients with acute coronary syndrome[19] and is the harbinger of multiplex proteomics diagnostic and prognostic assays to come.
Metabolic Profiling (Metabolomics). Another rapidly emerging technology is metabolomics. Metabolomics is the analysis of low molecular weight molecules involved in primary or intermediary metabolism and metabolites found in cells, tissues, and body fluids. The metabolome represents the culmination of all molecular events in a specific tissue -- gene variation and expression (genomics), protein expression, modification, and activity (proteomics), and environmental exposures.[21] A conservative estimate of the number of metabolites is 5000 compared with the more than 100,000 estimated protein species and more than 20,000 genes in humans. Because the metabolome represents an end stage of cell events, it may correlate with phenotype more directly.[20] However, this strength may also be a weakness because the metabolome is likely to be extremely dynamic and sensitive to a multitude of signals, disease-related and otherwise.
Single metabolite data are quite familiar to clinicians, with serum cholesterol and serum glucose as prime examples. Advances in high-throughput mass spectroscopy or nuclear magnetic resonance technologies allow detection, analysis, and identification of a range of metabolites (entire classes of small molecules, such as fatty acids, amino acids, nucleosides, and vitamins) that traditionally have been measured one at a time. The resulting metabolomic patterns are being developed as risk and monitoring markers.
Because all of these technologies are genome scale and high-throughput, patterns of biomarkers instead of measurements of single entities are becoming more commonplace. The benefit of detecting multiple measures of change is the ability to view the downstream biological events in aggregate, yielding a more complete picture of the disease potential, progression, or prognosis earlier in the process.
Next Page »
Last Shot Hydration Drink Announced as Official Sponsor of Red River Athletic Conference • EQLB • Jun 20, 2024 2:38 PM
ATWEC Announces Major Acquisition and Lays Out Strategic Growth Plans • ATWT • Jun 20, 2024 7:09 AM
North Bay Resources Announces Composite Assays of 0.53 and 0.44 Troy Ounces per Ton Gold in Trenches B + C at Fran Gold, British Columbia • NBRI • Jun 18, 2024 9:18 AM
VAYK Assembling New Management Team for $64 Billion Domestic Market • VAYK • Jun 18, 2024 9:00 AM
Fifty 1 Labs, Inc Announces Acquisition of Drago Knives, LLC • CAFI • Jun 18, 2024 8:45 AM
Hydromer Announces Attainment of ISO 13485 Certification • HYDI • Jun 17, 2024 9:22 AM