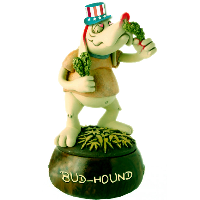
Thursday, December 18, 2008 3:06:28 AM
Slide 1: Case Study A Cancer Center Vision: The development and state-wide adoption of a centralized scientific and clinical Data Warehouse The Future of Personalized Medicine Edward Martinez (Former VP & CIO of Moffitt Cancer Center)
Slide 2: What is Personalized Medicine? “The use of biomarkers or molecular signatures to accelerate the development of new drugs targeted to specific subpopulations of patients.” -Former Director NCI
Slide 3: The Problem with Contemporary Cancer Care • Cancer is a complex, heterogeneous disease • Many patients are treated to help an unknown few — one size fits all • Drug therapy is generally not tailored to the patient • Response rates low, toxicity high • Patients do not want to leave home for treatment
Slide 4: Current Approach to Phase II Trials is Hit or Miss • Phase II trials test the efficacy (response rate) of a new drug for the first time • Patients recruited to trial on a first come first serve basis with no knowledge of target • Response to new drug “hit or miss” • 50% of Phase II drugs retired • Millions lost; 2 steps backwards
Slide 5: The Solution: Total Cancer Care Total Cancer Care Personalized Cancer Care 2010 Study large populations… Identify cancer sub-populations… Develop therapies for sub-populations of individuals…
Slide 6: Moffitt’s Total Cancer Care? • Perhaps the world’s largest translational research project • A means to collect, relate, and interpret clinical data and molecular data from thousands of patients across Florida • A mechanism to identify molecular signatures for diagnosis, prognosis, and prediction of response to therapy • A means to personalize cancer therapy by matching “pipeline” drugs to patients harboring molecular targets • A means to improve the quality of medicine
Slide 7: Hypothesis/Plan • Identify drug-specific molecular targets (or surrogate for it) • Determine prevalence of targets • Enrich pipeline drug trials with patients harboring the target • Engage and invest in community and academic partners in clinical trials and quality improvement • Develop and disseminate evidence based therapy standards • Decrease time to drug registration
Slide 8: New The Building Blocks of Total Standard Cancer Care Of Care Personalized Molecular Medicine Signatures Central Data Warehouse 50,000 Patients (Primaries + Metastatic Biopsies) QOL, Clinical Tissue Survivorship Surveys Banking Pipeline Evidence IT Quality Therapeutic Based Trials Solutions Medicine Improvement TCC Affiliate MCC Consortium Network (Resections + Trials) (Metastatic Biopsies)
Slide 9: TCC Mission & Value • Contribute to the cure of cancer • Identify the right drug for the right patient • Improve the performance of clinical trials • Measure and improve the quality of care • Bring clarity to and individual’s condition and treatment regimen • Raise the standard of care
Slide 10: State-wide affiliations allow for sharing of clinical and genomic information Potential to capture 50% of cancer cases In second highest cancer burden State
Slide 11: Strategic Approach • Use layered Plug ‘n’ Play approach • Build once and extend for other protocols • Enable rapid implementation • Develop seamless interconnection (clinical & research data sets) • Leverage existing systems, databases, applications and infrastructure • Enhance or expand systems as necessary • Incorporate industry standards (e.g. ca-BIG, HL7, CDISC) • Apply what we have learned from past experience
Slide 12: Data Warehouse Strategy • Design “Centralized” data Warehouse to answer questions for researchers, clinicians, bio- informatics personnel… • Develop seamless interconnection (clinical & research data sets)
Slide 13: Data Warehouse Design Objectives • Architecture – Built for rapid inflow of data – Data delivery accomplished via data marts, cubes… • Built to accept heterogeneous data sources and observations • Dynamically accounts for new data elements being added in source systems • Provides unified storage for questions that would span multiple operational systems • Association of research and clinical data from the enterprise • Data can be exported in multiple formats and shared with other systems as required
Slide 14: Operational Data Flow Challenges Capstone Cancer Registry MDDB Galvanon Capstone Colon 400 HPV PharmNet E Surginet T PathNet L Cerner ET Staging Area ETL SurgiNet L Data is extracted from source systems and delivered here Tissue Tracker DR ETL LabVantage OnCore Data Warehouse Micro Array Data is transformed into Standard Structures DM ETL Tissue Event EMR Genetic Data Analysis Analysis Analysis Analysis Mining Person Tissue Events EMR 14 Micro Array
Slide 15: Person-Centric Model Electronic Medical Surgical Data Survey Information Record • Procedures • Demographics • Treatment • Timelines • History • Medications • Screening • Lab results • Risk Assessment Cancer Registry Operational Data Person • Follow-up information • Billing • Diagnosis • Scheduling • Vital Status • Visit information Tissue Samples Clinical Trial Data MicroArray Data • Location • Protocols • Gene Expression • Gross/Tissue Dx • Studies • Experiment Analysis • Preparation • Participation • Sequence Verified • Type Annotation Linkage of Person to other data through a unique identifier 15 Independent of MRN, SS#, or any other known identifier
Slide 16: Data Elements – Sample Person Biospecimen EMR Trials Age Source Diagnosis Protocols Gender Category Lab Results Studies Race Gross Medications Phases Diagnosis Ethnicity Preparation Surgeries Participation Type Scheduling Consent Assay Results Physician Therapy Events MicroArray Questionnaire PHI Chip Type MRN Event Type Array Type Form SSN Hierarchy Probe set Observation Name Attribute Probe Answer Birth Date Result Signal Level Language Address Date Genes Present Skip Pattern Date of Death Association QC Metrics Phone 16
Slide 17: Pre- Warehouse • What patients have received chemotherapy? ● Cerner • How many lung tissue samples do we have? ● Tissue Tracker • Who is in this demographic? ● Galvanon • What gene’s are present or absent in this sample? ● Micro Array Data • Who is currently on this Clinical Trial? ● OnCore Queries are limited to a single system. Getting results that span more than one system requires extensive time and effort, and in some cases may not even be possible.
Slide 18: Post Warehouse • Find Caucasian females who have Breast Cancer, have received either chemotherapy or radiation and the number of tumor tissue samples we have ● (Data Warehouse) • For patients with lung cancer, show top 10 administered drugs and gene expression profile associated with those tumors for patients with survival greater than 24 months ● (Data Warehouse) • To qualify for a new clinical trial, show patients who have colon cancer, have smoked, have received a particular drug and have tissues samples available to study. ● (Data Warehouse) 18 Queries span multiple systems and provide a holistic view of data
Slide 19: Same Data – User Categories Nurse Bio-Informatics Scientist Data Manager Clinician Researcher
Slide 20: Classes of Data Users Highly Aggregated ● Aggregated Data ● Limited Drill Down ● Graphical Display, Dashboards, Interactive Executive More Detail User ● Standard & Ad hoc Reporting ● Parameter-Driven by Users at Run-Time ● Sorting, Selection, Filtering, Drill-Down ● Utilizing Standard Functions (e.g. calculations) Functional User Complete Raw Data Direct Access to Detailed Raw Data Power User ● ● “Just give me the data in SAS” ● Access-controlled Views
Slide 21: Vision: Aligned Data Sources Cerner OPTX Lawson Affiliate Systems Legacy Database Other Databases Tissue Bank Cancer Registry Sunquest Research CT Admin Systems
Slide 22: Vision: Disparate Sources CUI Interactive Presentation Common Data Semantics, Standards Extract, Transform, Load DB Lab or Medical Databases Other Systems Hand-Held IVR Instruments
Slide 23: Logical View: Multi-Dimensional Data Trial Descriptors Treatment Descriptors Site • Phase Descriptors • Protocol • Therapeutic Area • Type • Compound • Location • Sponsor • Contract Status • Protocol • IRB Facts Dimensions Integrated Clinical Clinical Data Data Mart Warehouse Physician / Investigator Detailed Clinical Data Facts Patient Descriptors Descriptors • Data on each Clinical Event • by Program • Demographics • Location • by Trial • Tx Group • Contract Status • by Site • Con Meds • Type • by Investigator • Prior Diagnoses • Fee Structure • by Patient • Outcomes
Slide 24: Logical View: Shared Meta-Data Patient Facts Descriptors Facts Dimensions Dimensions Data Data Warehouse Warehouse Location Descriptors Clinical Outcomes Facts Patient Satisfaction Facts Protocol Descriptors Facts Facts Investigator Dimensions Dimensions Descriptors Data Warehouse Data Warehouse Date / Time Trial Enrollment Facts Data Collection Facts Descriptors
Slide 25: Logical View: Process-Driven View Per Protocol Per Study Per Site Per Patient Per Visit Patient Patient Data Set Data Set Data Set Screened Randomized Received Coded Certified Data Set Queries CRFs CRFs Received Certified Patient Safety Patient on Listing Review Certified Patient Dropped Patient Withdrawn
Slide 26: Data Pipeline Source Data Data Preparation Data Provisioning Applications Trial Metrics Subject Area Data Marts Live (ad hoc) reports Master OpTx Extraction / Capture Reference Mapping Data (lists, code maps, dictionaries) Standard Reports Cerner M 01 Alerts F 02 IF(sum(a,b) . . . Cancer Registry Transformation Analytics / Dashboards Affiliate Systems Data Cubes
Slide 27: Enterprise Data Strategy Vision Requirements: Scientific, Business, Functional, Technical, Data Technology Architecture Implementation Plan
Slide 28: Data Exchange Interfaces
Slide 29: Data Warehouse Architecture Meta data Authentication & Authorization Domain - Role-based Access Control Cerner DM ` OpTx Client ETL Application Server Authentication & Authorization EAI (eGate/TDM) Data Access Layer DM ETL First Coast ` Client Data Staging Data ... Area Warehouse ` Client Application Server Source System n ` Secure HL7/ Client CDISC ODM ETL Affiliate source system 1 Non-EAI Affiliate Source source System system 2 Acquisition Storage Distribution Access & Presentation
Slide 30: Challenges • Initial investment required ($?) • Ability to support dynamically evolving requirements • Data integration effort across Moffitt and its affiliates • Process improvements required to support normalized data gathering efforts • Quality of existing data • Partnering with the right solution providers • Assessment of Affiliate technology capabilities to support strategic direction
Slide 31: Touch Screen Technology
Slide 32: Multi-Dimensional Clinical Data
Slide 33: Multi-Dimensional Clinical Data
Slide 34: Integrating Genomic and Clinical Data
Slide 35: Data Governance Executive Sponsors Data Governance and Monitoring Committee (DGMC) Data Data IT Owners Stewards Data Users
Slide 36: Data Governance Strategy • Fundamental Aspects of Data Generation & Data Consumption – Data is an Enterprise Asset – Authoritative Data Sources – Data Quality – Data Life Cycle – Data Ownership – Level of Aggregation – Protected Health Information (PHI) – Freezing of Data Sets & Versions of Data Release – Protection Against Misinterpretation
Slide 37: Phased Implementation OCT 2005 100 days FEB 2006 6 months AUG 2006 Ongoing Release 1 Release 3 Data Access Study 1 Clinical Trials Study 2 Clinical Trials Study 3 LIMS EMR Data Warehouse Data Warehouse Data Warehouse V0.1 V1.0 V1.1 Data Access Cancer Registry Intake Survey EMR: ● Registration ● Surgical Scheduling Specimen Bank ● Medications Summary ● Formulary Billing Surgery Affiliates Release 2 Microarray Data Access Partners
Slide 38: Three Portals to Data Patient View Clinician View Researcher View TCC Multi-Dimensional Data Warehouse View my lab Clinical Data Gene Expression Results online •Data guides Rx Clinical Data Signatures
Slide 39: Building on the Foundation Integrated Data System Meta data Authentication & Authorization Domain - Role-based Access Control Cerner DM ` OpTx Client ETL Application Server Authentication & Authorization Genomic Data EAI (eGate/TDM) Data Access Layer DM ETL First Coast ` Client Data Staging Data ... Area Warehouse ` Client Application Server Source System n ` Biospecimen Data Secure HL7/ Client CDISC ODM ETL Affiliate source system 1 Non-EAI Affiliate Source source System system 2 Acquisition Storage Distribution Access & Presentation Quality P4P Risk Factors Costs
Slide 40: How Pharmacogenomics Can Streamline Clinical Trials & Build Pharma Partnerships Traditional Clinical TCC Trial Trials Responders Only Broad Patient Population 10-12 Years 3-5 Years
Slide 41: Trial Enrichment 300 patients profiled in database • New Phase II opened in Florida Network •30 patients identified & matched to new Phase II trial • Trial completed in record time • Drug has significant chance for POS, less toxicity
Slide 42: PLASMA EXPRESSION URINE PROTEOMICS PROFILNG PROTEOMICS (PREOP/postop) BIOINFORMATICS CONSULTING COMMERCIAL OPPORTUNITIES CPG CGH/LOH METHYLATION AGILENT/ (AGILENT AFFY SNP CHIP BASED) M2GEN TCC DATA WAREHOUSE TISSUE SINGLE CELL PHOSPHO- SEQUENCING PROTEOMICS (SOMATIC (SHOTGUN) MUTATIONS) PARAFFIN Molecular RTPCR Imaging HIGH THROUGHPUT TMA IN HOUSE VS. TECHNOLOGIES OUTSOURCE
Slide 43: Lessons Learned to Date • QI projects are feasible at affiliate sites • Patients of all ages can participate in web based surveys • Local physician leadership and buy in key • Centralized TCC consenting likely more effective than distributed model • Don’t affect physicians workload or patient flow • Develop value for patients, physicians, hospitals, funding partners
Slide 44: Quotes from: The Council of Scientific Advisors, 2003 “This extraordinary initiative serves dual purposes: utilizing clinical expertise of the Moffitt Cancer Center for the good of cancer patients throughout Florida; and creating a network that, if properly utilized, could improve the quality of care and provide enormous opportunities for health outcomes research including research in quality of care and cost-effectiveness.” David Brailer, MD – President’s Consultant on Health Information Systems, 2004 “This needs to happen, and it needs to happen (in Florida).” Anne Barker, MD, Deputy Director of NCI, 2005 “You have just seen a glimpse into the future of medicine, and the way it needs to be.”
Slide 45: Edward Martinez emartinez33@hotmail.com 813-420-4703
VAYK Exited Caribbean Investments for $320,000 Profit • VAYK • Jun 27, 2024 9:00 AM
North Bay Resources Announces Successful Flotation Cell Test at Bishop Gold Mill, Inyo County, California • NBRI • Jun 27, 2024 9:00 AM
Branded Legacy, Inc. and Hemp Emu Announce Strategic Partnership to Enhance CBD Product Manufacturing • BLEG • Jun 27, 2024 8:30 AM
POET Wins "Best Optical AI Solution" in 2024 AI Breakthrough Awards Program • POET • Jun 26, 2024 10:09 AM
HealthLynked Promotes Bill Crupi to Chief Operating Officer • HLYK • Jun 26, 2024 8:00 AM
Bantec's Howco Short Term Department of Defense Contract Wins Will Exceed $1,100,000 for the current Quarter • BANT • Jun 25, 2024 10:00 AM