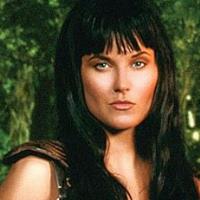
Thursday, July 14, 2016 10:01:18 PM
Towards Vision-Based Deep Reinforcement Learning for Robotic Motion Control
Fangyi Zhang, Jürgen Leitner, Michael Milford, Ben Upcroft, Peter Corke
(Submitted on 12 Nov 2015 (v1), last revised 13 Nov 2015 (this version, v2))
This paper introduces a machine learning based system for controlling a robotic manipulator with visual perception only. The capability to autonomously learn robot controllers solely from raw-pixel images and without any prior knowledge of configuration is shown for the first time. We build upon the success of recent deep reinforcement learning and develop a system for learning target reaching with a three-joint robot manipulator using external visual observation. A Deep Q Network (DQN) was demonstrated to perform target reaching after training in simulation. Transferring the network to real hardware and real observation in a naive approach failed, but experiments show that the network works when replacing camera images with synthetic images.
Comments: 8 pages, to appear in the proceedings of Australasian Conference on Robotics and Automation (ACRA) 2015
Subjects: Learning (cs.LG); Computer Vision and Pattern Recognition (cs.CV); Robotics (cs.RO)
Cite as: arXiv:1511.03791 [cs.LG]
(or arXiv:1511.03791v2 [cs.LG] for this version)
Submission history
From: Fangyi Zhang [view email]
[v1] Thu, 12 Nov 2015 06:19:59 GMT (4489kb,D)
[v2] Fri, 13 Nov 2015 05:41:08 GMT (4489kb,D)
Which authors of this paper are endorsers? | Disable MathJax (What is MathJax?)
http://arxiv.org/abs/1511.03791
SafePath navigation could be applied to the arms of this unit and they would not fail....
VHAI - Vocodia Partners with Leading Political Super PACs to Revolutionize Fundraising Efforts • VHAI • Sep 19, 2024 11:48 AM
Dear Cashmere Group Holding Co. AKA Swifty Global Signs Binding Letter of Intent to be Acquired by Signing Day Sports • DRCR • Sep 19, 2024 10:26 AM
HealthLynked Launches Virtual Urgent Care Through Partnership with Lyric Health. • HLYK • Sep 19, 2024 8:00 AM
Element79 Gold Corp. Appoints Kevin Arias as Advisor to the Board of Directors, Strengthening Strategic Leadership • ELMGF • Sep 18, 2024 10:29 AM
Mawson Finland Limited Further Expands the Known Mineralized Zones at Rajapalot: Palokas step-out drills 7 metres @ 9.1 g/t gold & 706 ppm cobalt • MFL • Sep 17, 2024 9:02 AM
PickleJar Announces Integration With OptCulture to Deliver Holistic Fan Experiences at Venue Point of Sale • PKLE • Sep 17, 2024 8:00 AM